The Future Of LLM Siri: Apple's Challenges And Solutions
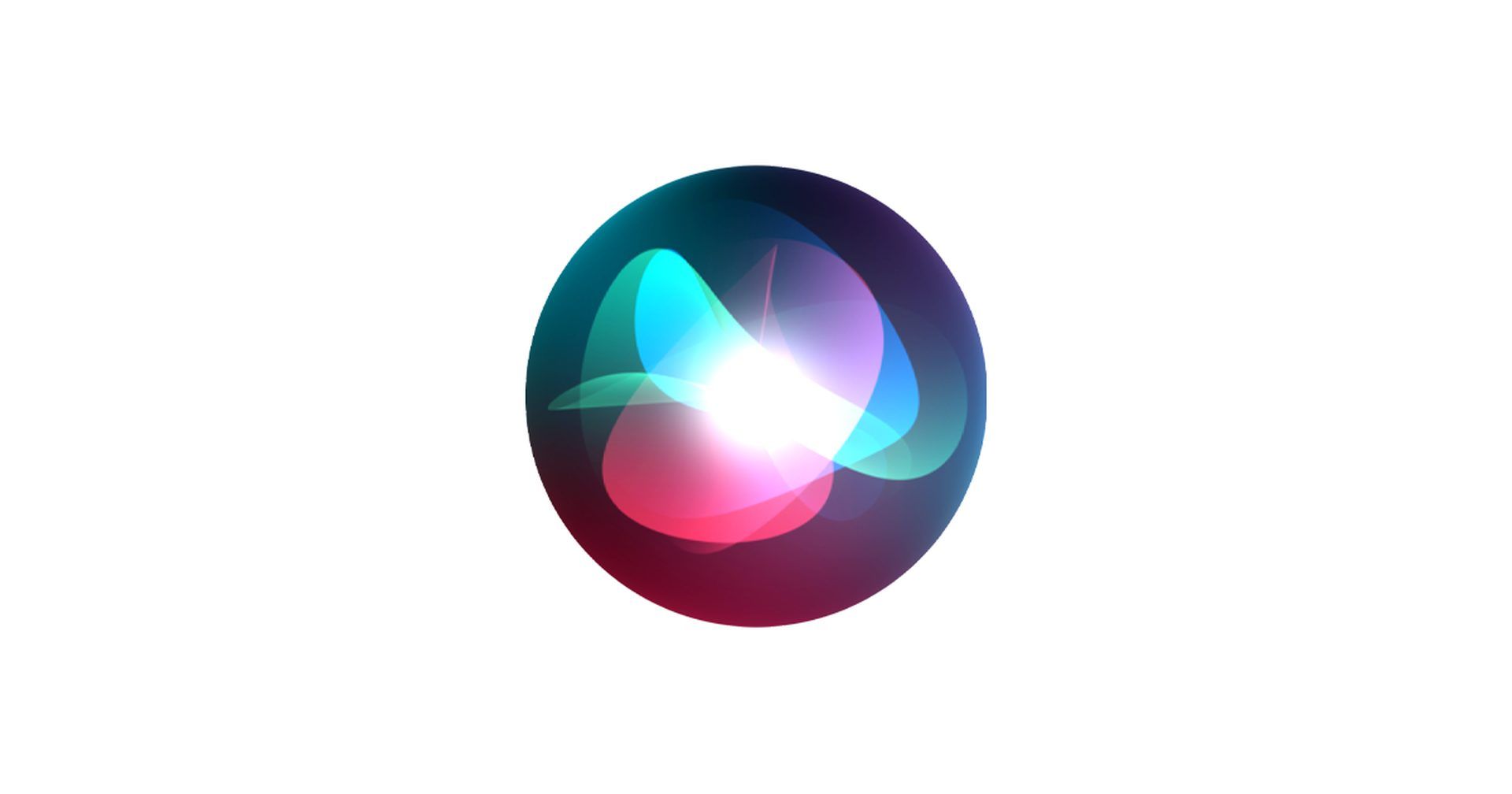
Table of Contents
The Current State of Siri: Falling Behind the Competition
Siri, while functional, lags behind competitors in several key areas. Its current capabilities are limited compared to the advanced natural language processing (NLP) demonstrated by LLMs integrated into other voice assistants. This gap impacts user experience and satisfaction. Siri struggles with complex requests, often providing inaccurate or irrelevant responses. Its contextual understanding is also lacking, leading to frustrating interactions.
- Limited contextual understanding: Siri often fails to grasp the context of a conversation, requiring users to repeat information or rephrase requests.
- Difficulty handling complex requests: Multi-step instructions or nuanced queries often overwhelm Siri's capabilities.
- Weak integration with other Apple services and third-party apps: Seamless integration with the Apple ecosystem is crucial for a superior user experience, and Siri falls short in this area.
- Inferior natural language processing compared to LLMs: Siri's NLP capabilities are demonstrably less sophisticated than those powered by LLMs, resulting in less accurate and less helpful responses. This is a key area where Siri needs significant improvement to compete effectively.
Key Challenges Apple Faces in Integrating LLMs into Siri
Integrating LLMs into Siri presents Apple with several significant hurdles. Addressing these challenges is crucial for the success of future LLM Siri iterations.
Data Privacy Concerns
Apple has built its brand on a strong commitment to user privacy. This presents a significant challenge when training LLMs, which typically require vast datasets for optimal performance.
- Balancing data usage for model improvement with user privacy: Apple must find a way to leverage user data for training without compromising privacy. This necessitates innovative approaches to data anonymization and aggregation.
- Developing privacy-preserving LLM training techniques: Federated learning and differential privacy are promising techniques that could allow Apple to train LLMs without directly accessing sensitive user data.
- Addressing potential biases in training data while minimizing data collection: LLMs trained on biased data can perpetuate harmful stereotypes. Apple must develop robust methods for identifying and mitigating bias while minimizing the amount of data collected.
Computational Resources and Energy Efficiency
LLMs are computationally intensive, requiring significant processing power and energy. This poses a challenge for mobile devices like iPhones and iPads, where power efficiency is paramount.
- Optimizing LLM models for Apple's hardware: Apple needs to develop LLMs specifically tailored for its hardware, optimizing performance while minimizing power consumption.
- Developing efficient on-device processing capabilities: Processing requests entirely on the device would improve speed and privacy, but requires significant advancements in on-device processing capabilities.
- Exploring federated learning approaches for improved efficiency: Federated learning can distribute the computational load across multiple devices, reducing the burden on any single device.
Maintaining Siri's User-Friendly Interface
Siri's success is partly due to its intuitive and user-friendly interface. Integrating complex LLM functionalities without compromising this simplicity is a key challenge.
- Designing intuitive interfaces for complex LLM functionalities: Apple needs to design interfaces that make advanced LLM capabilities accessible to users of all technical skill levels.
- Balancing advanced capabilities with simple user interactions: The design needs to avoid overwhelming users with complex options while still providing access to powerful features.
- Ensuring seamless integration with existing Siri features: New LLM functionalities must integrate smoothly with existing features to maintain a consistent and familiar user experience.
Potential Solutions for Apple to Enhance LLM Siri
Several strategies can help Apple overcome these challenges and enhance LLM Siri.
Investment in Advanced LLM Research and Development
Significant investment in R&D is crucial for developing LLMs specifically optimized for Siri.
- Acquiring or partnering with leading LLM companies: Collaborations with established players could accelerate development and access cutting-edge technology.
- Building internal expertise in LLM development: Investing in hiring top talent and fostering internal research is essential for long-term success.
- Focusing on LLMs optimized for mobile devices: LLMs tailored for mobile devices will offer improved performance and energy efficiency.
Improved Data Annotation and Model Training
Improving the quality and quantity of training data is essential for improving Siri's accuracy and performance.
- Utilizing user feedback to improve model accuracy: Collecting user feedback on Siri's responses can help identify areas for improvement and refine the model.
- Implementing advanced data augmentation techniques: Data augmentation can increase the size and diversity of the training dataset, leading to a more robust model.
- Developing more robust methods for handling noisy or incomplete data: Real-world data is often noisy or incomplete. Apple needs to develop robust methods for handling this to ensure model accuracy.
Enhanced Integration with Apple Ecosystem
Deeper integration with the Apple ecosystem is key to unlocking LLM Siri's full potential.
- Enabling more contextual and personalized responses: Access to user data (while respecting privacy) can lead to more relevant and personalized responses.
- Expanding Siri's capabilities across different Apple devices: Seamless functionality across iPhones, iPads, Macs, and Apple Watches enhances user experience.
- Creating seamless workflows between Siri and other apps: Integration with other apps allows users to accomplish tasks more efficiently.
Conclusion
The future of LLM Siri depends on Apple's ability to address the challenges of data privacy, computational resources, and user interface design. By investing heavily in research and development, improving data annotation and model training, and enhancing ecosystem integration, Apple can revitalize Siri and position LLM Siri as a leading voice assistant. The potential for a more intelligent, capable, and privacy-respecting Siri is immense. Don't miss out on the exciting developments – stay tuned for the next iteration of LLM Siri and the innovations to come!
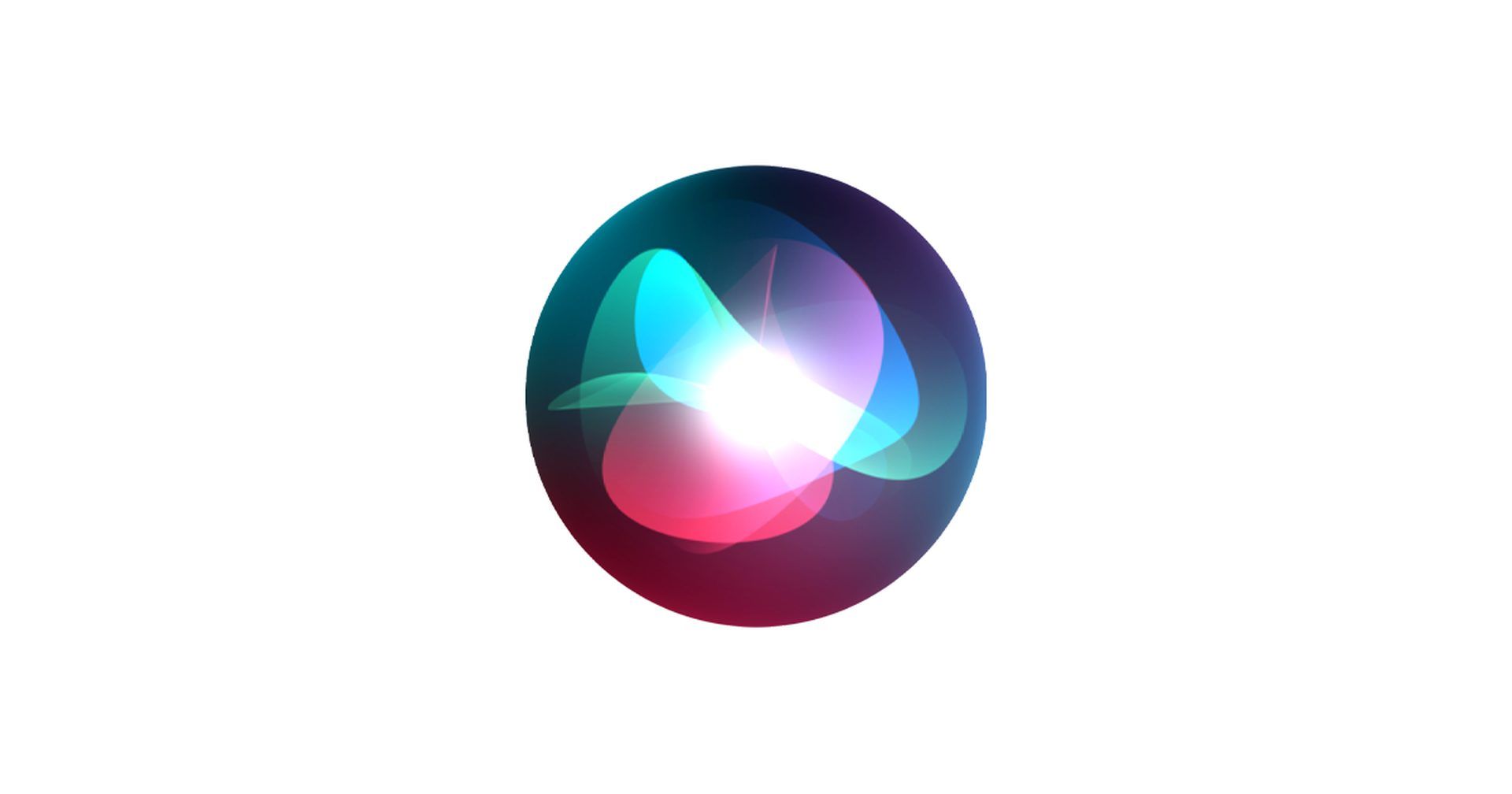
Featured Posts
-
Cronin Appointed Highfield Rugby Head Coach
May 20, 2025 -
Nyt Crossword April 25 2025 Find The Answers Here
May 20, 2025 -
The Impact Of Brexit On Uk Luxury Exports To The Eu
May 20, 2025 -
F1 Kaoset Detaljerad Analys Av Hamilton Och Leclercs Diskvalifikation
May 20, 2025 -
Investing In Big Bear Ai Bbai Is This Ai Penny Stock A Smart Buy
May 20, 2025
Latest Posts
-
Understanding Winter Weather Advisories And School Closings
May 20, 2025 -
Your First Alert Strong Wind And Severe Storms Expected
May 20, 2025 -
School Delays Due To Winter Weather Advisory
May 20, 2025 -
This Ai Quantum Computing Stock A Dip Buying Opportunity
May 20, 2025 -
Winter Weather Advisory School Delays And Closures
May 20, 2025