Turning "Poop" Into Podcast Gold: An AI-Powered Approach To Repetitive Documents
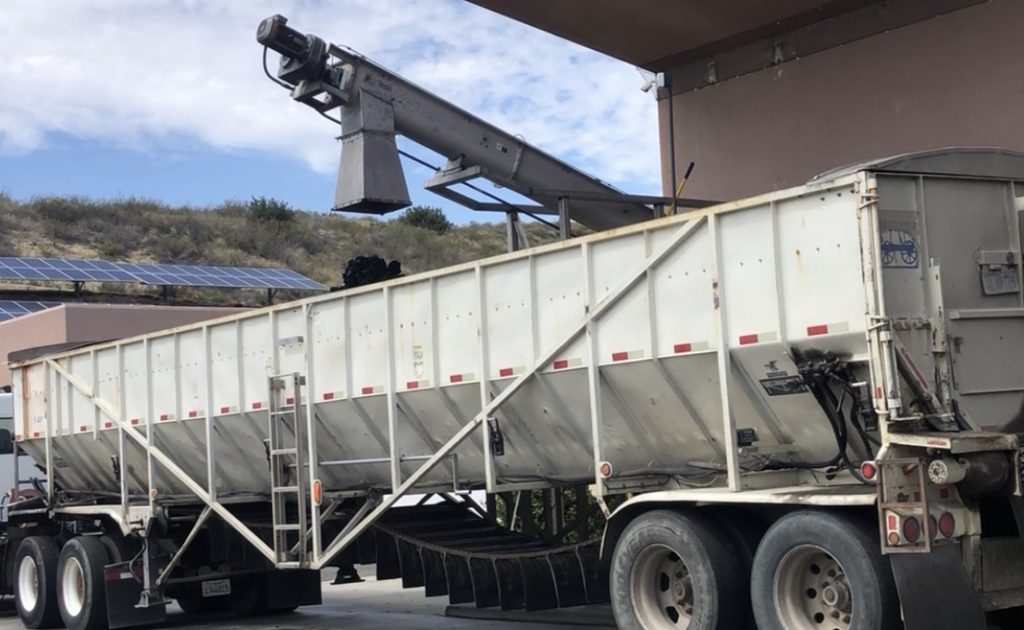
Table of Contents
Identifying and Quantifying Repetitive Documents
Dealing with data redundancy is a common problem across various industries. Before implementing any AI solution, it's crucial to first identify and quantify the extent of the problem. This allows for a focused and effective approach.
Recognizing Redundancy Patterns
AI algorithms are incredibly effective at identifying duplicated or near-duplicate content within large datasets. This goes beyond simple exact matches; sophisticated techniques can uncover subtle similarities.
-
Keyword Focus: Data redundancy, duplicate content detection, near-duplicate detection, document similarity analysis.
-
Bullet Points:
- Natural Language Processing (NLP): NLP techniques analyze textual similarity, identifying paraphrased sentences, similar phrasing, and semantic equivalence even when the wording isn't identical.
- Machine Learning Models: These models excel at pattern recognition in numerical data, identifying recurring structures and anomalies indicative of redundancy.
- Similarity Metrics: Techniques like Jaccard similarity (measuring the overlap of sets) and cosine similarity (measuring the angle between vectors representing documents) provide quantitative measures of document similarity. These help determine the degree of redundancy.
Measuring the Impact of Redundancy
Once you've identified redundant documents, it's time to quantify the impact. This provides a clear picture of the potential benefits of using AI to streamline your processes.
-
Keyword Focus: Efficiency gains, cost-benefit analysis, ROI (Return on Investment), time savings.
-
Bullet Points:
- Percentage of Redundant Data: Calculate the percentage of your entire dataset comprised of redundant information. This provides a clear baseline.
- Time Savings Estimation: Project the amount of time saved by automating tasks that currently involve processing redundant documents.
- Cost Savings Projection: Estimate the cost reductions achieved by reducing manual labor and improving efficiency. This helps demonstrate the ROI of implementing AI solutions.
AI-Powered Solutions for Handling Repetitive Documents
The true power of AI in tackling data redundancy lies in its ability to automate tasks and improve data management.
Automation of Data Entry and Extraction
Manual data entry from repetitive documents is slow, error-prone, and expensive. AI automates this process dramatically.
-
Keyword Focus: Data extraction, automation, AI-powered data entry, robotic process automation (RPA).
-
Bullet Points:
- Optical Character Recognition (OCR): OCR converts scanned documents (paper or images) into digital text, making them searchable and processable by AI.
- Natural Language Processing (NLP): NLP extracts key information from unstructured text, such as identifying dates, names, and amounts within invoices or contracts.
- Machine Learning for Classification: Machine learning algorithms can categorize and sort documents based on their content, streamlining workflow and improving searchability. This is particularly useful for large document repositories.
AI-Driven Document Summarization and Consolidation
AI doesn't just automate; it also helps create more manageable and efficient document sets.
-
Keyword Focus: Document summarization, AI-powered summarization, content consolidation, data reduction.
-
Bullet Points:
- Extractive Summarization: This method selects the most important sentences from a document to create a concise summary.
- Abstractive Summarization: A more advanced technique, this creates entirely new summaries that capture the essence of the original document.
- Keyword Extraction: This helps identify the key topics and themes within a set of similar documents, further aiding in consolidation and analysis.
Real-World Examples and Case Studies
The benefits of AI in handling repetitive documents are demonstrable across numerous sectors.
-
Keyword Focus: Case studies, success stories, real-world applications, industry examples.
-
Bullet Points:
- Law Firm: A law firm might use AI to analyze thousands of similar legal documents, quickly identifying key clauses and precedents, significantly reducing research time.
- Financial Institution: Financial institutions can automate data entry from invoices and receipts, minimizing errors and improving reconciliation processes.
- Research Institution: Researchers can use AI to consolidate research papers on a specific topic, creating comprehensive summaries and identifying gaps in existing knowledge.
Conclusion
Transforming repetitive documents from a tedious burden to a valuable asset is now possible thanks to AI. By identifying and quantifying data redundancy and employing AI-powered solutions, you can significantly improve efficiency, reduce costs, and unlock new opportunities. Don't let repetitive documents bog you down – embrace AI and start turning your "poop" (data redundancy) into podcast gold (valuable insights and productivity). Start exploring AI-powered document processing solutions today to experience the benefits firsthand.
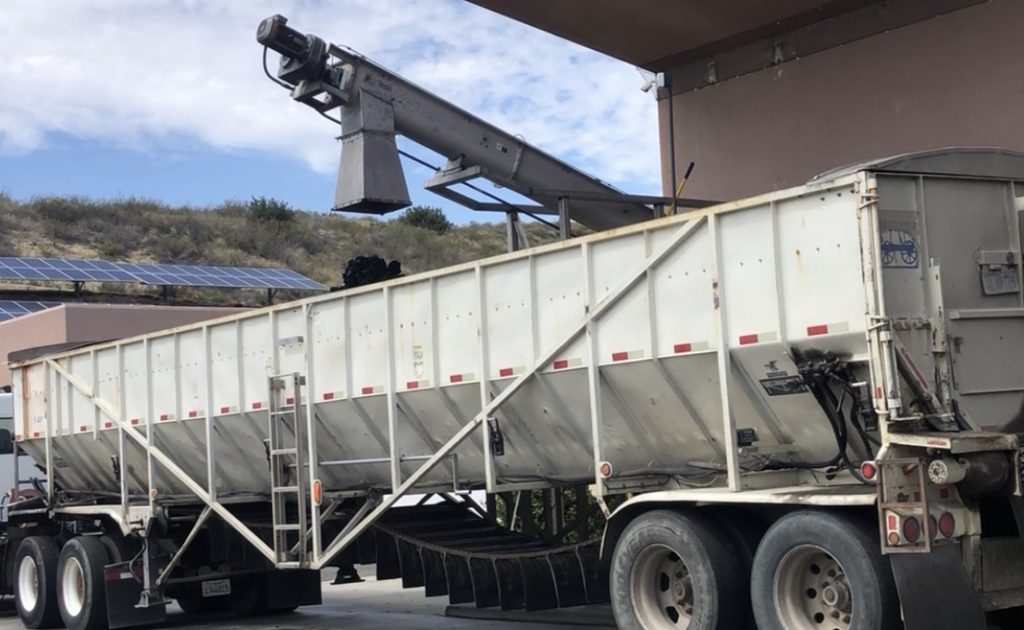
Featured Posts
-
Anti Trump Protests A Cross Country Perspective
Apr 22, 2025 -
Us Protests Against Trump Voices From Across The Nation
Apr 22, 2025 -
Ftc To Appeal Activision Blizzard Acquisition Decision
Apr 22, 2025 -
Chainalysis Acquires Alterya Blockchain Meets Ai
Apr 22, 2025 -
Overcoming The Hurdles Automating Nike Sneaker Manufacturing
Apr 22, 2025