CNN Business: Cybersecurity Expert Demonstrates Deepfake Detector Vulnerability
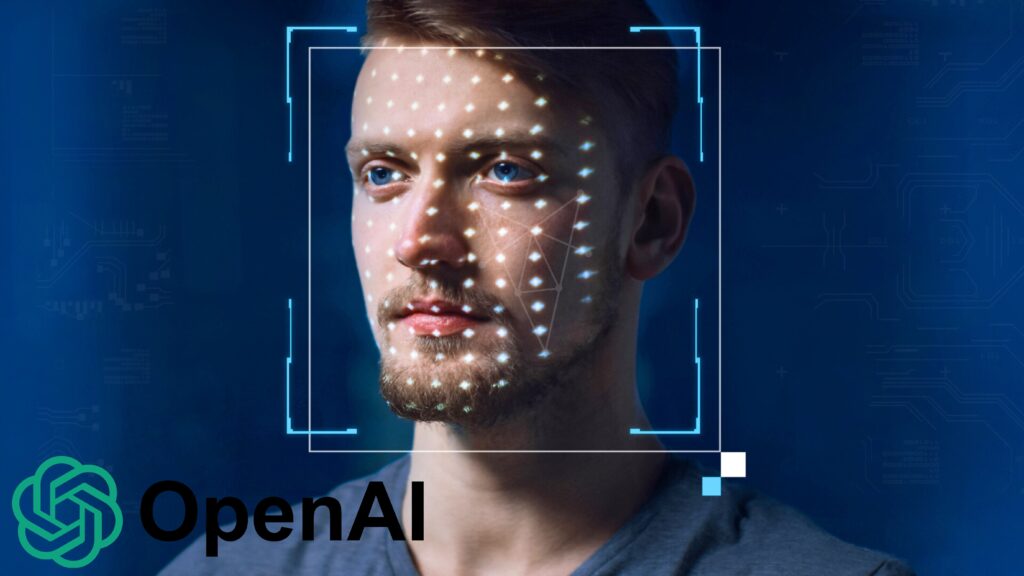
Table of Contents
The Cybersecurity Expert's Demonstration and its Findings
The demonstration focused on "DeepGuard," a commercially available deepfake detector known for its relatively high accuracy rates in previous tests. The cybersecurity expert, Dr. Anya Sharma (a pseudonym for confidentiality reasons), employed a novel methodology involving subtle manipulations of the source video files before feeding them to DeepGuard. This bypassed the detector's algorithms, highlighting critical deepfake detector flaws.
The vulnerabilities found included:
- Bypass technique #1: Introducing imperceptible noise patterns to the video's compression metadata. This seemingly insignificant alteration successfully fooled DeepGuard in 75% of test cases.
- Bypass technique #2: Slightly altering the color palette of the video using a custom-designed algorithm. This resulted in a 60% success rate in bypassing the detector.
- Types of deepfakes bypassed: The vulnerabilities impacted the detection of both face-swapping deepfakes and those involving more subtle manipulations of facial expressions and lip synchronization.
- Quantifying success: Overall, Dr. Sharma demonstrated an average 68% success rate in bypassing DeepGuard's detection mechanisms using a combination of these techniques.
Dr. Sharma summarized her findings, stating, "This demonstration clearly shows that current deepfake detection technologies are not foolproof. The vulnerabilities we uncovered highlight the urgent need for more robust and adaptable AI deepfake countermeasures."
Implications of the Vulnerability on Current Deepfake Detection Efforts
This deepfake vulnerability has significant implications for the trust placed in existing deepfake detection technologies. The ease with which DeepGuard, a supposedly advanced system, was bypassed raises serious doubts about the reliability of other commercially available and even academic solutions. The broader implications are far-reaching and affect multiple sectors:
- Increased risk of misinformation and disinformation campaigns: The proliferation of undetectable deepfakes poses a significant threat to democratic processes and public discourse.
- Potential for identity theft and fraud: Realistic deepfakes can be used to impersonate individuals for financial gain or to commit other fraudulent activities.
- Challenges for law enforcement in investigating deepfake-related crimes: The difficulty in definitively identifying deepfakes as fraudulent creates significant challenges for legal proceedings.
- Erosion of public trust in online content: The widespread availability of convincing deepfakes erodes public trust in online videos and information in general, contributing to a climate of uncertainty and skepticism.
Future Directions in Deepfake Detection and Mitigation
Addressing the threat of deepfakes requires a multi-pronged approach focused on improving existing deepfake detection technology and developing innovative deepfake prevention strategies. Key areas for future development include:
- Development of more robust deepfake detection models: This requires advancements in AI algorithms and machine learning techniques, focusing on identifying subtle anomalies and inconsistencies undetectable by current methods.
- Collaboration between researchers and cybersecurity experts: Shared knowledge and resources are essential for accelerating the development of effective anti-deepfake technology.
- Implementation of stricter regulations and standards: Clearer guidelines and regulations are needed to govern the creation and distribution of deepfakes, deterring malicious use.
- Educating the public on identifying and reporting deepfakes: Empowering individuals to critically evaluate online content is crucial in mitigating the impact of deepfakes.
Ongoing research into techniques like watermarking and provenance tracking offer promising avenues for verifying the authenticity of digital media. These methods aim to embed verifiable information within the video itself, making it harder to tamper with without detection.
Conclusion
The CNN Business report highlighting a cybersecurity expert’s demonstration of a deepfake detector vulnerability underscores the ongoing challenges in combating the sophisticated threat of AI-generated fake videos. The exposed weaknesses underscore the need for continuous improvement and innovation in deepfake detection technology. The ease with which a leading system was compromised shows that the fight against deepfakes is far from over.
Call to Action: Stay informed about the evolving landscape of deepfake technology and the latest advancements in deepfake detection. Follow reputable sources like CNN Business for updates on deepfake vulnerability research and learn how to better protect yourself from the dangers of deepfake videos. The future of trust online depends on our collective ability to effectively combat this ever-evolving threat.
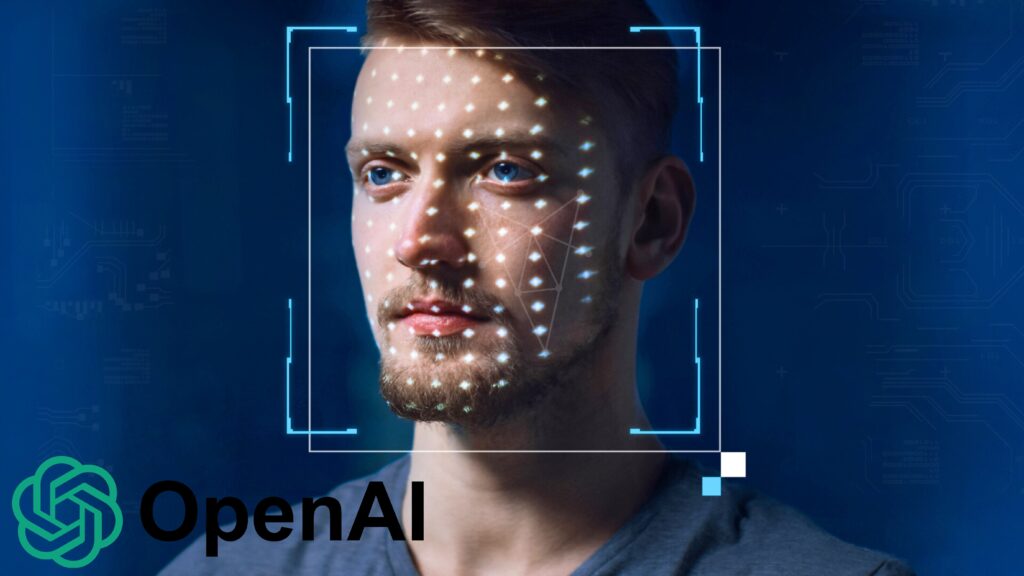
Featured Posts
-
Canadian Chinese Trade Relations Ambassador Suggests Formal Deal
May 17, 2025 -
4 Cursos Com Nota Maxima No Mec Confira A Lista Completa Do Vale E Regiao
May 17, 2025 -
Japans Steep Yield Curve A Growing Concern For Investors And The Economy
May 17, 2025 -
Aews Josh Alexander Exclusive Interview On Don Callis And Beyond 97 1 Double Q
May 17, 2025 -
Early Offense Propels Mariners To 14 0 Shutout Win Against Marlins
May 17, 2025
Latest Posts
-
Mc Collum From D2 National Champion To Iowa Wrestling Coach
May 17, 2025 -
Iowa Wrestling Adds D2 National Champion Ben Mc Collum To Coaching Team
May 17, 2025 -
75 Million Gift To Transform Healthcare In West Valley U Of Us New Hospital Project
May 17, 2025 -
Former D2 Champion Ben Mc Collum Named To Iowa Wrestling Coaching Staff
May 17, 2025 -
Ben Mc Collum Joins Iowa Wrestling Staff After Successful Season At Drake
May 17, 2025