Improving The Accuracy Of Automated Visual Inspection For Lyophilized Vials
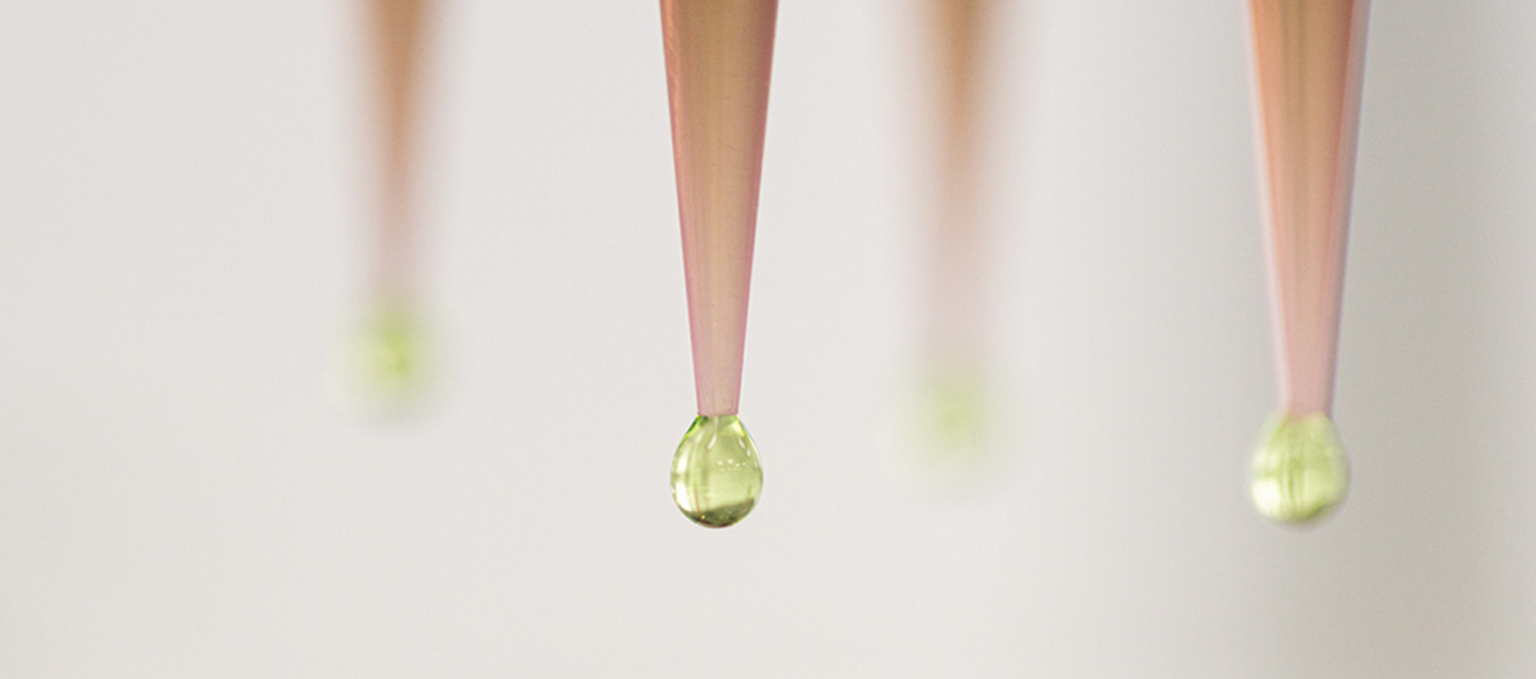
Table of Contents
Enhancing Image Acquisition Techniques for Improved Accuracy
High-quality images are fundamental to accurate automated visual inspection. Optimizing image acquisition directly impacts the system's ability to detect even subtle defects in lyophilized vials.
Optimizing Lighting and Camera Settings
Proper lighting is crucial for highlighting surface imperfections. The choice between diffuse and direct lighting, along with the spectral characteristics of the light source, significantly affects image contrast and defect visibility.
- Lighting Techniques:
- Coaxial Lighting: Provides even illumination, minimizing shadows and enhancing the visibility of surface defects like cracks.
- Ring Lighting: Offers uniform illumination around the vial, useful for detecting particulate matter.
- Darkfield Lighting: Ideal for highlighting scratches and other surface irregularities by creating a high contrast against a dark background.
Camera parameters are equally important. Higher resolution cameras capture finer details, allowing for the detection of smaller defects. Proper exposure time prevents over- or under-exposure, ensuring optimal image brightness and contrast. The choice of sensor type (e.g., CMOS vs. CCD) also impacts image quality, noise levels, and sensitivity.
- Camera Parameter Optimization:
- Resolution: Higher resolution (megapixels) improves the detection of small cracks and particulate matter.
- Exposure Time: Correct exposure prevents washed-out images (overexposure) or images too dark to analyze (underexposure).
- Sensor Type: CMOS sensors are generally more cost-effective, while CCD sensors often provide higher image quality and lower noise.
Advanced Imaging Modalities
Beyond standard RGB imaging, advanced techniques offer significant advantages in detecting subtle defects often missed by conventional systems.
- Hyperspectral Imaging: Captures images across a wide range of wavelengths, revealing information about the vial's material composition and enabling the detection of defects based on spectral signatures. This is particularly useful for identifying subtle variations in the lyophilized product itself.
- Multispectral Imaging: Similar to hyperspectral, but utilizes a smaller number of specific wavelengths, offering a balance between information richness and processing speed. This can be highly effective in detecting delamination or inconsistencies in the lyophilization process.
- 3D Imaging: Provides a three-dimensional representation of the vial, allowing for the detection of defects such as vial collapse or uneven fill levels that might be missed with 2D imaging.
These advanced modalities significantly enhance the sensitivity and accuracy of automated visual inspection systems for lyophilized vials, improving overall quality control.
Implementing Robust Image Processing and Analysis Algorithms
Sophisticated image processing algorithms are essential for accurately identifying and classifying defects in the acquired images.
Defect Detection Algorithms
Several algorithms are employed for automated defect detection in lyophilized vials. The choice depends on the type of defects to be detected and the available computational resources.
- Machine Learning Algorithms: Techniques like Support Vector Machines (SVMs) and Random Forests can be trained to classify defects based on features extracted from the images.
- Deep Learning Algorithms: Convolutional Neural Networks (CNNs) are particularly well-suited for image analysis, achieving high accuracy in detecting complex patterns and subtle defects. They are ideal for detecting cracks, particulate matter, and delamination.
Training these algorithms requires a substantial dataset of labeled images representing various defects and non-defective vials. The quality and size of this training dataset significantly impact the algorithm's performance.
False Positive and False Negative Reduction
Minimizing errors in defect classification is critical for ensuring the reliability of automated visual inspection.
- Reducing False Positives: Techniques like adaptive thresholding, morphological filtering, and background subtraction can effectively remove noise and reduce false positive classifications.
- Reducing False Negatives: Ensemble methods (combining multiple algorithms) and data augmentation (increasing training data variability) can significantly improve the sensitivity of the system to detect subtle defects, reducing false negatives.
Improving the Overall System Design and Workflow
The overall system design and workflow significantly influence the effectiveness of automated visual inspection.
Automated Vial Handling and Presentation
Efficient and reliable vial handling is critical to ensuring consistent image acquisition and preventing damage to the vials.
- Automated Vial Handling Systems: Robotic arms and conveyor belts are commonly used to automate vial transport and presentation to the imaging system.
- Minimizing Vial Orientation Variations: Precise vial orientation is crucial to ensure consistent image acquisition and prevent misinterpretations by the defect detection algorithms. This requires robust mechanical design and precise control systems.
Integration with Existing Quality Control Systems
Seamless integration with existing quality control systems is crucial for improving traceability and reporting.
- Data Integration Strategies: Automated systems should be able to exchange data with existing systems using standard formats like CSV, XML, or direct database connections.
- Enterprise Resource Planning (ERP) Integration: Integrating the automated visual inspection system with ERP systems allows for comprehensive data management, traceability, and reporting, enhancing overall quality control and regulatory compliance.
Conclusion
Improving the accuracy of Automated Visual Inspection for Lyophilized Vials involves a multi-faceted approach encompassing optimized image acquisition, robust image processing algorithms, and a well-designed system workflow. By optimizing lighting and camera settings, employing advanced imaging modalities, implementing effective defect detection algorithms, and minimizing false positives and negatives, manufacturers can achieve significantly higher accuracy in identifying defects. Furthermore, integrating these systems with existing quality control workflows enhances overall efficiency and regulatory compliance. Investing in and implementing these techniques will lead to reduced waste, increased throughput, improved product quality, and enhanced confidence in the integrity of lyophilized vials. Consider exploring and implementing these strategies to optimize your automated visual inspection processes and achieve superior results in your lyophilized vial inspection protocols. Transitioning to advanced automated visual inspection systems is a key step toward achieving consistent, high-quality pharmaceutical production.
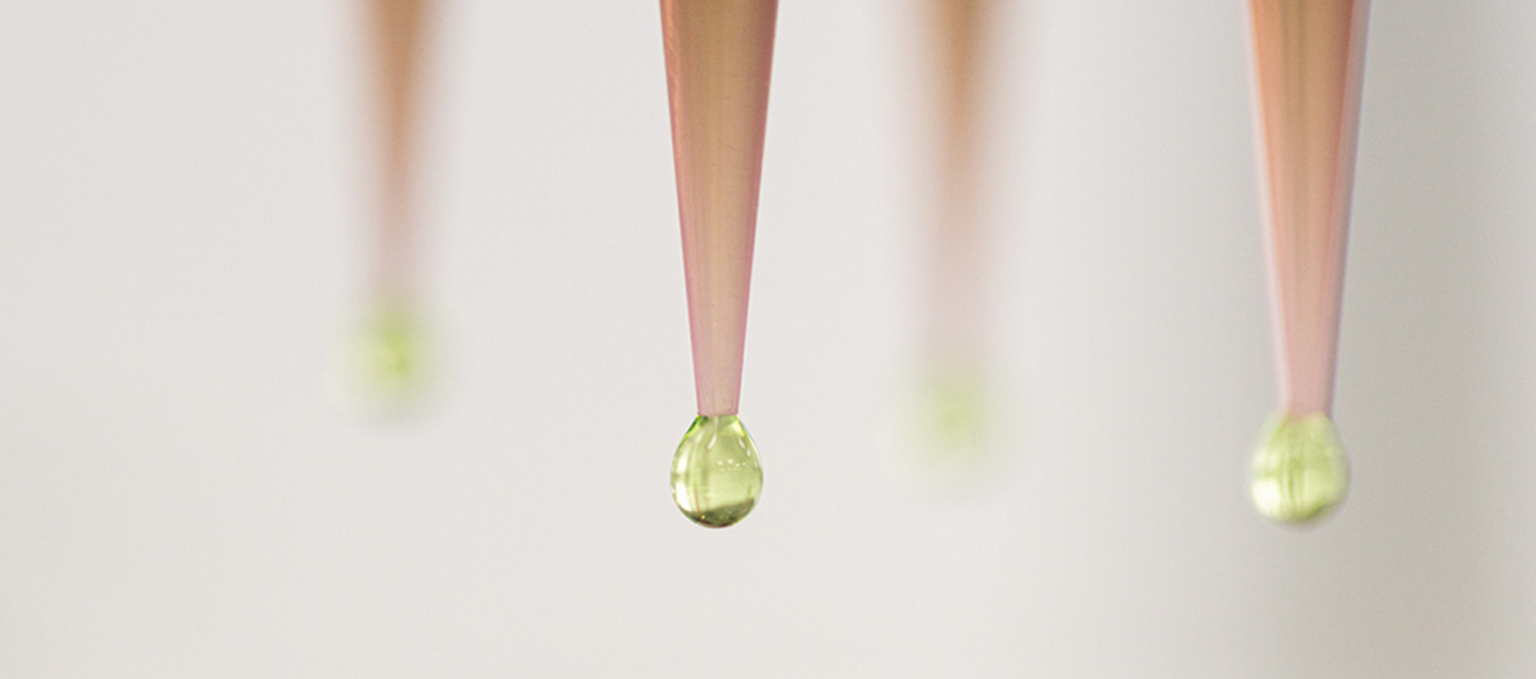
Featured Posts
-
Bmw And Porsche In China Market Headwinds And Strategic Responses
May 11, 2025 -
The Ultimate Guide To Mtv Cribs Best Mansions
May 11, 2025 -
Suri Cruises Birth Tom Cruises Reaction And Subsequent Actions
May 11, 2025 -
How Instagram Plans To Compete With Tik Tok Ceos Testimony Highlights Key Strategies
May 11, 2025 -
Next Papal Conclave Potential Candidates For The Papacy
May 11, 2025