Podcast Production: How AI Processes Repetitive Scatological Data
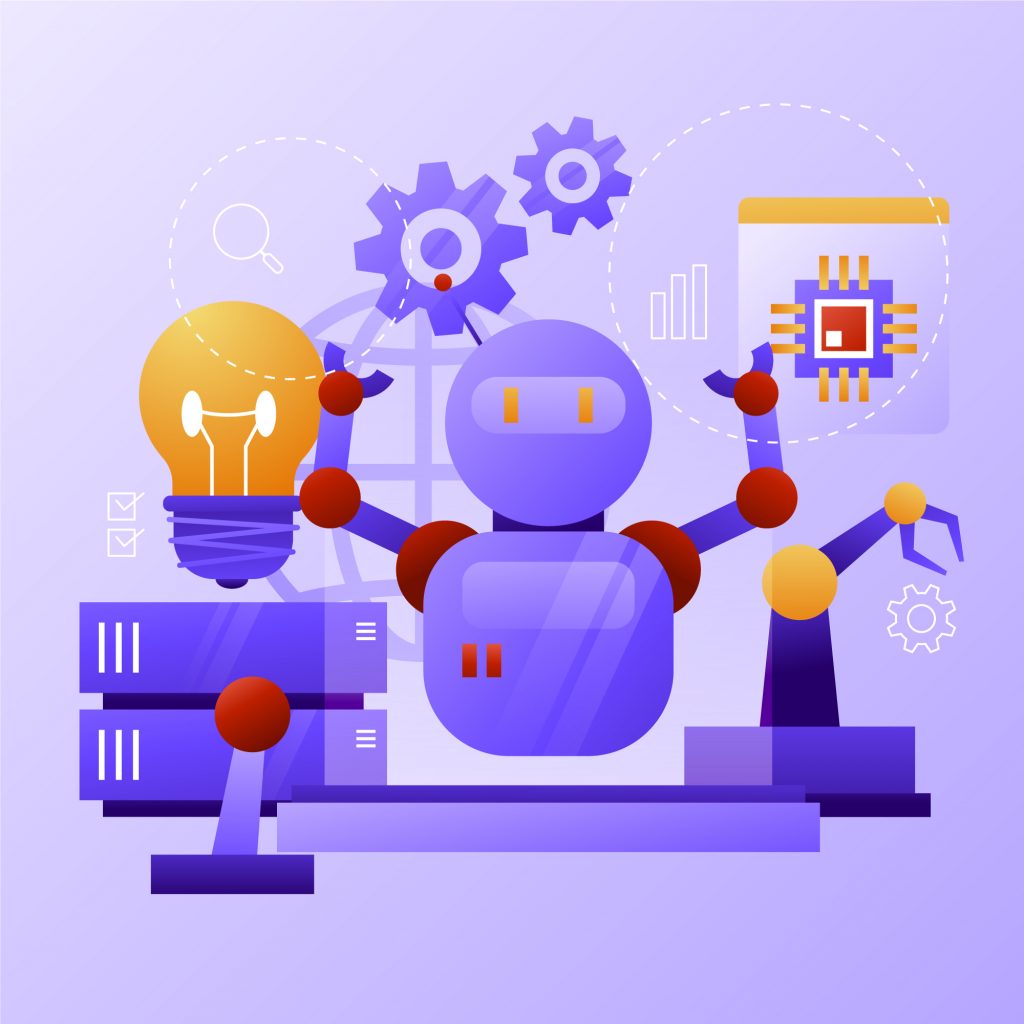
Table of Contents
Identifying and Removing Unwanted Sounds with AI
Podcasters often face the challenge of unwanted sounds in their recordings. These can range from minor distractions to more significant issues, sometimes including sounds that fall into the scatological category. AI is proving invaluable in addressing this problem.
Automated Scatological Sound Detection
AI algorithms are trained to recognize and isolate specific sounds, including those considered scatological, which are often unintentionally recorded during podcast creation. This sophisticated technology goes beyond simple noise reduction; it's about intelligent identification of specific, unwanted audio.
- Improved accuracy: AI significantly reduces human error in identifying these sounds compared to manual methods. Manual cleaning is time-consuming and prone to mistakes, especially when dealing with subtle or nuanced sounds.
- Time savings: Automated detection speeds up the editing process substantially. This allows podcasters to spend more time on creative aspects and less time on tedious cleanup.
- Examples: Unexpected coughs, sneezes, background noises (like a running toilet or other undesirable sounds), or even unintentional profanity can be automatically flagged for review or removal. This targeted approach ensures only the problematic audio is addressed.
Contextual Understanding for Precise Editing
Advanced AI systems go beyond simple sound detection; they incorporate contextual understanding. This means the AI can differentiate between a relevant discussion about bodily functions (perhaps a medical podcast) and an accidental recording of a toilet flush.
- Reduced risk of content loss: Contextual understanding prevents the removal of vital podcast segments. The AI understands the nuance of the conversation and avoids deleting important information.
- Enhanced editing precision: AI ensures only truly irrelevant scatological audio is removed, maintaining the integrity of the podcast's content. This level of precision is difficult, if not impossible, to achieve through manual editing.
AI-Powered Noise Reduction and Enhancement
Beyond identifying and removing specific sounds, AI plays a crucial role in enhancing the overall audio quality of a podcast. This includes minimizing background noise and optimizing audio levels.
Minimizing Background Noise
AI algorithms effectively reduce background noise and interference, creating a cleaner, more professional-sounding podcast. This includes reducing the impact of environmental noises that might be considered scatological in nature, improving listener experience.
- Improved audio clarity: Listeners experience a better listening experience due to reduced distractions. Clear audio significantly improves listener engagement and retention.
- Professional sound quality: Enhances the overall production value of the podcast, making it sound more polished and credible. This is essential for attracting and retaining a larger audience.
Automated Audio Equalization and Compression
AI can automatically adjust audio levels, improving consistency and clarity across the podcast. This includes minimizing harsh or unpleasant sounds that could be categorized as scatological, ensuring a balanced and pleasant listening experience.
- Consistent audio quality: Maintains consistent volume levels throughout the recording, preventing jarring jumps in loudness.
- Optimized audio dynamics: Enhances the overall listening experience by ensuring the audio is neither too quiet nor too loud. This leads to a more enjoyable and less fatiguing listening experience.
The Future of AI in Podcast Scatological Data Processing
The application of AI in podcast audio processing is constantly evolving. We can expect even more sophisticated tools in the future.
- Real-time processing: Future AI tools may offer real-time audio cleanup during recording, eliminating post-production headaches. This would streamline the entire workflow significantly.
- Personalized settings: AI systems could learn individual preferences, allowing for customized processing of scatological and other unwanted sounds. This ensures the AI adapts to the unique needs and preferences of each podcaster.
- Integration with existing DAWs: Seamless integration with popular Digital Audio Workstations (DAWs) will make AI tools easily accessible to podcasters, simplifying the process of incorporating this technology into existing workflows.
Conclusion
AI is transforming podcast production by efficiently managing the often-overlooked aspect of repetitive and scatological data processing. Through automated detection, noise reduction, and enhancement, AI frees podcasters to focus on creating engaging content, ultimately improving the quality and efficiency of their workflow. By adopting AI-powered tools, podcast creators can significantly improve their audio quality, saving valuable time and resources. Don't let unwanted sounds, including scatological data, bog down your podcast production. Embrace the power of AI today and elevate your podcast to the next level with advanced audio processing.
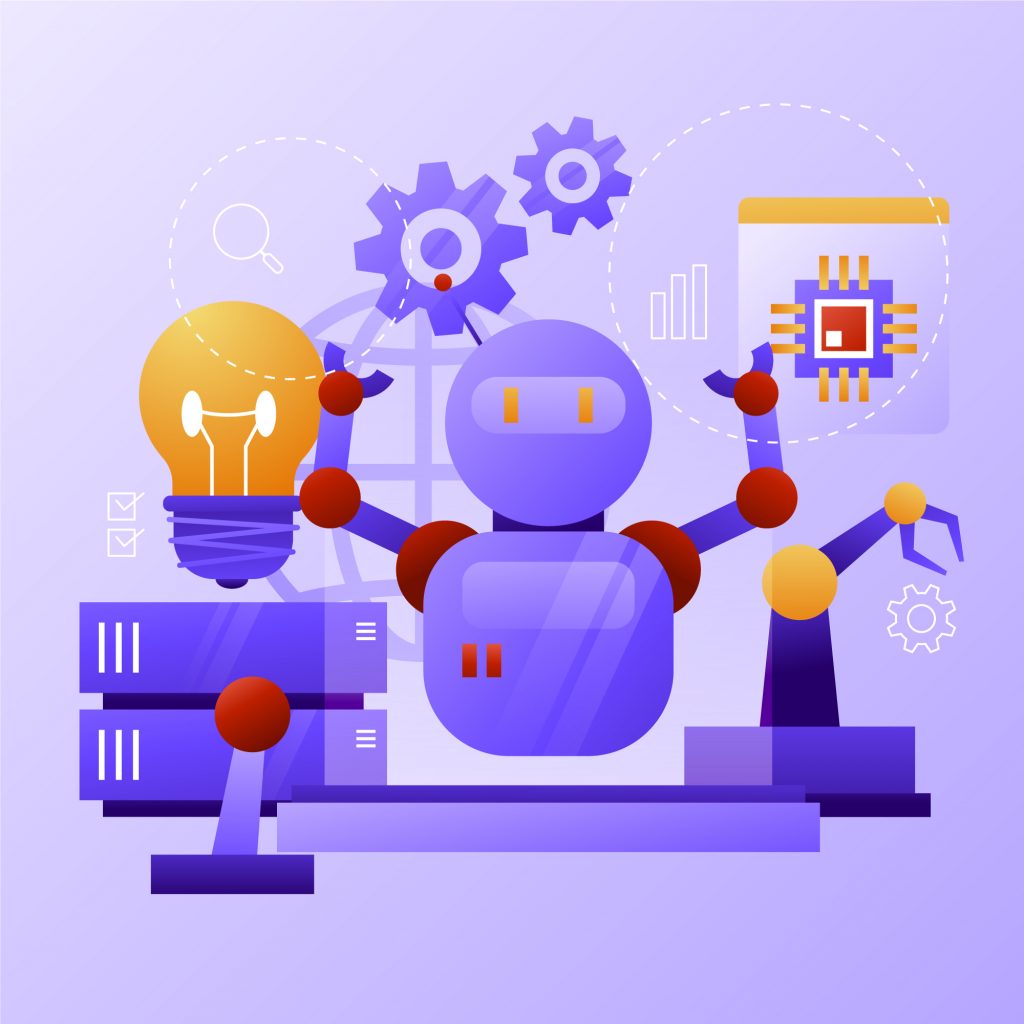
Featured Posts
-
Im Convinced Star Wars Will Finally Show Us This Long Teasd Planet
May 17, 2025 -
Josh Harts Wifes Reaction To Jaylen Browns Game 5 Performance
May 17, 2025 -
Seattle Mariners Pitcher Bryce Miller On 15 Day Il Elbow Injury
May 17, 2025 -
Us Tariffs Reshape Honda Production A Canadian Advantage
May 17, 2025 -
All Conference Honors A Track Roundup Of Top Athletes
May 17, 2025