The Role Of AI In Protecting Endangered Species: A Critical Analysis
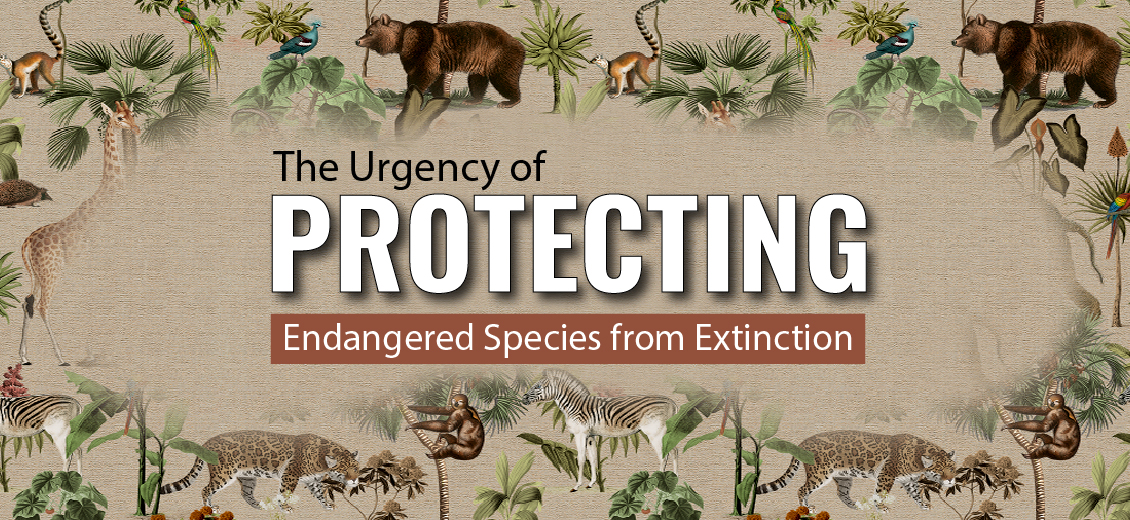
Table of Contents
AI-Powered Monitoring and Surveillance
AI is revolutionizing how we monitor endangered species and their habitats. Sophisticated technologies are providing unprecedented insights into the lives of these vulnerable populations, leading to more effective conservation strategies.
Real-time Tracking and Habitat Monitoring
AI-powered drones, equipped with high-resolution cameras and thermal imaging, can cover vast areas, providing real-time data on species locations and movements. Satellite imagery analysis, coupled with machine learning algorithms, allows for large-scale habitat monitoring, identifying deforestation, poaching activities, and habitat fragmentation. Sensor networks deployed in critical habitats can collect data on various environmental parameters, providing a comprehensive understanding of the species' environment.
- Successful Applications: AI has successfully tracked elephant poaching in real-time, leading to quicker interventions and arrests. Similarly, AI-powered systems have been used to monitor whale migration patterns, informing the creation of protected marine areas.
- Challenges: Processing and analyzing the massive datasets generated by these technologies require significant computing power and expertise. Accuracy can also be affected by factors like weather conditions, vegetation density, and the limitations of sensor technology. Furthermore, deploying and maintaining the necessary infrastructure in remote areas can be logistically challenging and expensive.
Automated Species Identification and Classification
AI algorithms, particularly image recognition and acoustic analysis, are automating the identification and classification of species from various data sources. Camera traps, deployed across habitats, generate thousands of images, which can be efficiently analyzed by AI to identify different species, even in challenging conditions. Similarly, AI can analyze audio recordings to identify species based on their unique vocalizations.
- Improved Accuracy and Speed: AI significantly improves the accuracy and speed of species identification compared to manual methods, allowing for faster responses to threats such as habitat loss or poaching.
- Challenges: AI algorithms are susceptible to biases in the training datasets, potentially leading to inaccurate classifications. They also struggle with camouflaged or partially visible species, requiring ongoing refinement and improvements. Furthermore, large, high-quality training datasets are necessary for optimal performance, requiring significant investment in data collection and annotation.
Predictive Modeling and Risk Assessment
AI's ability to analyze complex datasets and identify patterns enables predictive modeling, a crucial tool for proactive conservation. By anticipating threats, conservationists can deploy resources more effectively and implement targeted interventions.
Forecasting Population Trends and Habitat Change
AI models can analyze historical and current data on various factors, including climate data, habitat loss rates, human activity, and population density, to predict future population trends. This allows for proactive conservation strategies, such as targeted habitat restoration or translocation programs.
- Proactive Conservation: Predictive models enable conservationists to anticipate potential threats and take preventative measures, maximizing the effectiveness of resource allocation.
- Challenges: The accuracy of predictions depends heavily on the quality and completeness of the input data. Complex models can also be difficult to interpret and validate, requiring expert knowledge to ensure their reliability.
Identifying Poaching Hotspots and Illegal Wildlife Trade Networks
AI can analyze crime data, such as seizure records, arrest locations, and trafficking routes, to identify patterns and predict future poaching events. This information can then be used to inform law enforcement strategies, leading to more targeted interventions and a reduction in illegal wildlife activities.
- Improved Law Enforcement: AI-driven predictive policing can help law enforcement agencies focus their resources on high-risk areas, improving the efficiency of anti-poaching efforts.
- Challenges: Data privacy concerns must be addressed when using sensitive law enforcement data for AI modeling. Effective collaboration between law enforcement agencies and AI developers is crucial for successful implementation.
AI-Driven Conservation Strategies and Decision-Making
AI is not just a monitoring tool; it is rapidly becoming a crucial component of conservation strategy development and decision-making.
Optimizing Conservation Efforts Through Data Analysis
AI tools can analyze massive datasets to identify the most effective conservation strategies. This could involve optimizing habitat restoration projects, prioritizing areas for conservation efforts, or improving the design of translocation programs.
- Cost-Effective Solutions: AI can help maximize the impact of limited resources by identifying the most efficient and cost-effective conservation approaches.
- Challenges: Integrating AI insights into existing conservation frameworks and decision-making processes can be challenging, requiring collaboration between scientists, policymakers, and conservation practitioners.
Citizen Science and Public Engagement
AI is making it easier for the public to participate in conservation efforts. AI-powered image analysis apps, for example, allow citizens to contribute to data collection by identifying species from photos they take.
- Increased Public Participation: Citizen science initiatives fueled by AI increase the volume and geographical reach of data collection, improving the scope and accuracy of conservation monitoring.
- Challenges: Ensuring the quality and accuracy of data contributed by non-expert citizens requires careful quality control mechanisms and user education.
Conclusion
AI offers significant potential for revolutionizing endangered species protection by providing efficient monitoring tools, predictive capabilities, and data-driven decision-making support. However, it's crucial to acknowledge the limitations and potential challenges associated with implementing AI-based conservation strategies. Responsible AI development, addressing ethical considerations such as data privacy and bias, is paramount. The future of endangered species conservation hinges on our ability to harness the power of AI responsibly. Join the movement to protect our planet's biodiversity by learning more about the role of AI in endangered species protection and supporting initiatives focused on its ethical and effective implementation.
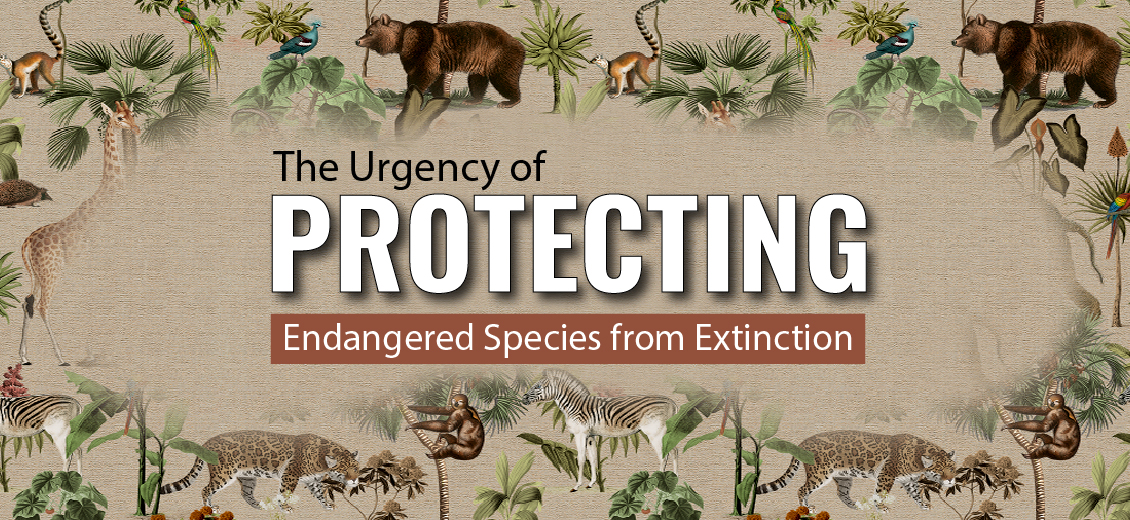
Featured Posts
-
Okullar Pazartesi Tatil Mi Istanbul Da Guencel Bilgiler
Apr 23, 2025 -
Market Analysis Dow Futures Gold Prices And Current Economic Uncertainty
Apr 23, 2025 -
Poilievres Electoral Setback A Deep Dive Into Lost Support
Apr 23, 2025 -
Todays Market Dow Futures Gold Prices And Key Economic Indicators
Apr 23, 2025 -
Another Win For Sf Giants Flores And Lees Crucial Contributions
Apr 23, 2025